Data-driven decision making
How to make data more trustworthy and valuable
You probably have a love/hate relationship with data: you know you need it and you take advantage of opportunities to collect and use it; but you don’t completely trust it. You’re right: data is the weak link in achieving a thorough analysis of any problem or decision. Data-driven decision making begins with trust in the data.
Data becomes more trustworthy and valuable when you:
- Understand the types of analysis
- Align your data to your goals
- Ask the right people for information
- Make sure you can access and understand the data
- Apply the data to create long-lasting change.
Trust in data depends on understanding the types of analysis
A descriptive analysis tells you what has happened in the past; a predictive analysis gives insight into the future. A diagnostic analysis looks for explanations of what happened in the past; and a prescriptive analysis suggests the best course of future action.
For example, a descriptive analysis might tell you that returns of your product have increased 25% over the last six months. A predictive analysis would indicate that, if returns continue to increase at that rate, customer satisfaction will drop precipitously and demand for your products will decrease. A diagnostic analysis might show that all the returns are related to a specific product with quality issues.
When you depend on a purely descriptive analysis without diagnostics, you might correct the wrong problem. In the example, you might tighten up your returns policy rather than investigating quality issues. You might blame your quality assurance team for poor performance when, in fact, the quality issue began with a change in raw materials.
The value of data depends on your goals
An experienced, reputable excavation company fell further and further behind a years-long project with no idea why. They assumed the problem stemmed from poor management. The never looked at their data to confirm that assumption.
A global manufacturing company had issues delivering on time, in full (OTIF). They, too, blamed the problem on poor management, believing that the warehouse team was not pulling its weight. Yet the company had no metrics to support that belief, no effective sales, inventory, and operations planning process (SIOP), and no executive visibility into the supply chain.
In both cases, the companies tried numerous remedies but based those remedies on a preconceived notion: fixing poor management would fix the problem. They never looked at their own data to determine the root causes of the problems.
The data revealed that, in the case of the excavation company, excavators and dozers were breaking down regularly and often sitting idle waiting for fuel, repairs, and other contractors to finish their work. They needed to improve asset availability and utilization.
The manufacturing company’s lack of metrics aligned with their customer satisfaction goals meant that executives lacked visibility into how their own supply chain worked. Once they had standardized data across the supply chain, root cause analysis revealed that the company’s downstream problems began upstream.
Data-driven decisions are only achievable if you:
- Align the metrics you use with your goals.
- Apply those metrics across the plan-buy-make-move supply chain
- Avoid assumptions and look for root causes in the data.
The trustworthiness of data depends on who you ask for it
A maker of satellite platforms suffered from increased competition, tighter budgets, and extreme internal finger-pointing. If information were gathered from only engineering or only procurement or only operations, three very different solutions would have emerged. The key to fixing the culture and the problems was to gather data from everyone across planning, finance, sales, procurement, operations, and logistics.
With clear communication, engineering made sure that specifications had the clarity and detail that manufacturing needed. Everyone collaborated together to move from a “make first” mentality to an understanding of what was core to manufacturing, secondary, and non-core. A new role was created to enhance communications between procurement, manufacturing, and engineering. The result included $68 million in annualized savings.
Data-driven decision making requires that you:
- Accept input from every function in the supply chain, including procurement, operations, and logistics.
- Build collaboration with your suppliers and your customers for a free flow of information.
- Standardize the data, so that everyone is using the same terms and applying the same metrics.
Trustworthy data is accessible and understandable
You can’t achieve data-driven insights if the data is too difficult to find and understand, regardless of whether it is accurate, timely, and complete. For example, a buying organization was losing both members and suppliers and did not know why. The data they needed was buried in mountains of manually processed invoices.
Using A.I. to plow through 40 GB of invoices from over 200 manufacturers, the company uncovered buying trends for the first time and compared supplier performance and prices. Structured data fields provided SKU-level, line item visibility, and an executive dashboard clearly identified profit drivers. Plus the system allowed them to add new invoices as they arrived, delivering timely as well as accessible data. In addition, a group purchasing management operating system also give insight into member behavior for the first time.
Data-driven depends depend on whether you:
- Organize the data in a way that makes sense for the people using the data.
- Install a system for visualizing data.
The value of data increases when it is actionable
You analyze data to change outcomes: to stop projects from falling behind, meet customer expectations, improve profits, free up working capital, and so on. Data analytics might provide information on:
- Asset availability and utilization
- Capacity and capability planning
- Cost of goods sold
- Distribution planning
- Energy management and carbon reduction
- Footprint optimization
- Forecasting consumer demand
- Obsolescence and waste management
- Performance
- Pricing
- Receivables management
- Should cost
- SKU rationalization
- Spend
- Supplier optionality.
Working on and with all that data at the same time is a lose-lose proposition; it is too overwhelming. That is why you need specific a goal, metrics associated with that goal, and a clear understanding of what the data means before you take any action.
Trustworthy, timely, complete data is the basis for any type of action, whatever the type of analytics: descriptive, predictive, exploratory, or diagnostic. Once you take action, you must continue to evaluate the data. If you implement changes, does the data change? If not, what are the roadblocks? Have you engaged the right people in finding and implementing change? Does everyone know their owner-responsible-consult-inform (ORCI) role and accountability?
Quality, trustworthy data leads to quality, data-driven decision making.
Related Resources
Ready to take hold of your supplier performance management?
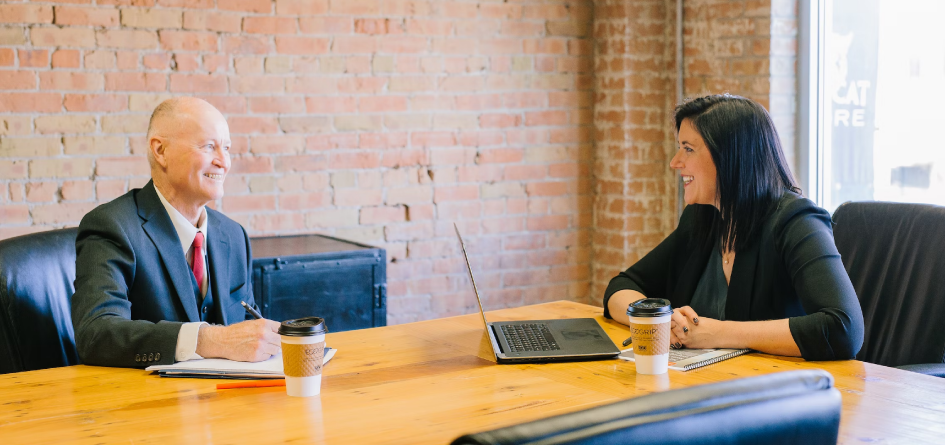
Talk to us
From rapid sprints for short-term gains to transformation for competitive advantage we are here to get you there.
Schedule a discussion
Submit this form to speak to an SGS Maine Pointe representative.
Prefer a call? (781) 934 - 5569